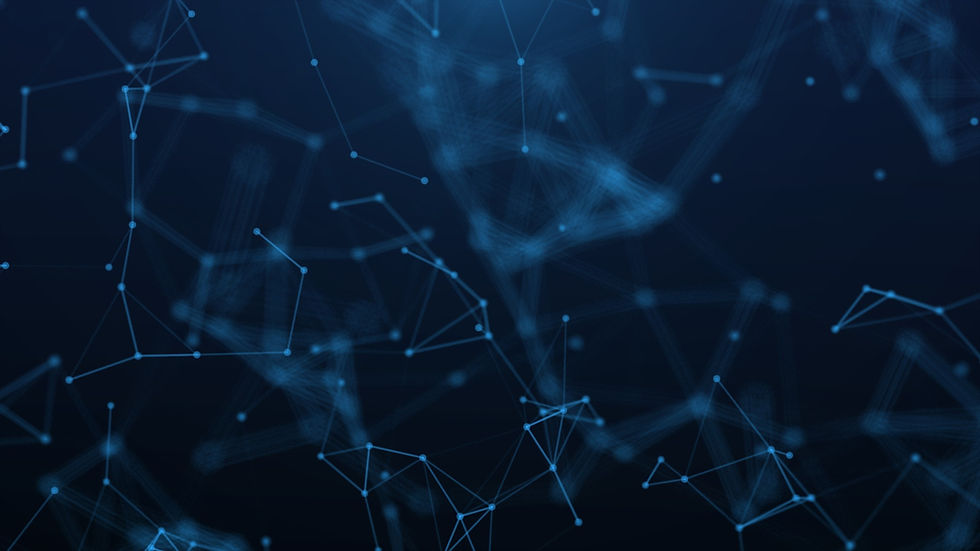
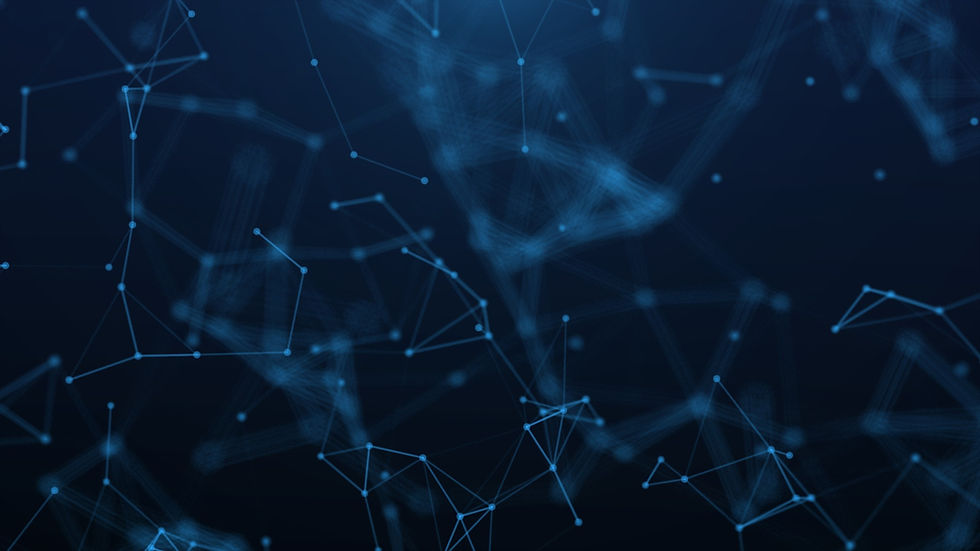
AnalyTEAcs'
Automated Channel Selection
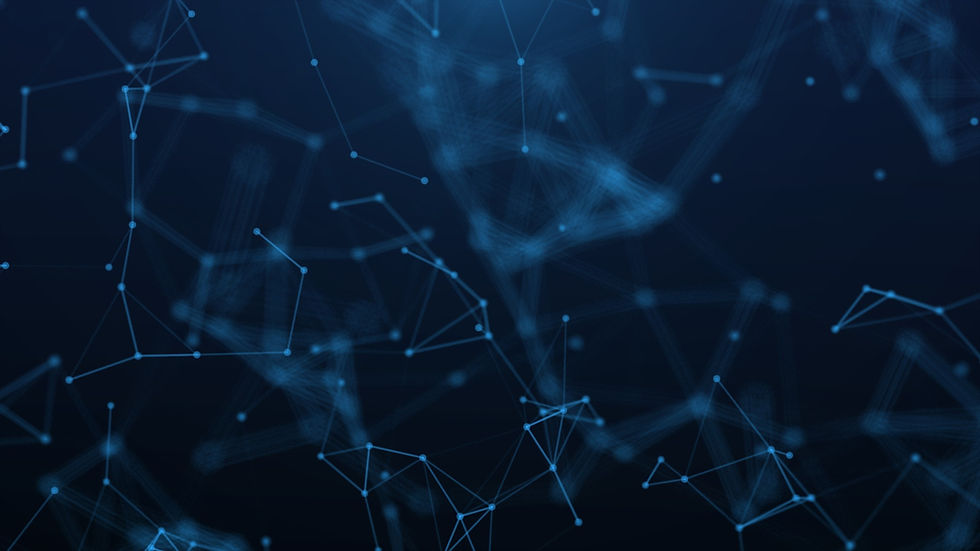
Abstract
​
This whitepaper by TEA Networks tackles the prevalent challenges in wireless mesh networks (WMNs) such as interference and inefficient channel utilization, which significantly impact their performance, security, and scalability. It introduces the Automated Channel Selection feature, an innovative approach that integrates predictive analytics and machine learning algorithms, optimized with Apache Spark, to enhance network efficiency and reliability. This solution methodically anticipates potential interference, facilitating strategic channel selection to improve network performance. It equips network administrators with periodic, actionable insights, enabling informed decision-making for channel optimization. By addressing these critical network management challenges, the Automated Channel Selection feature sets the stage for WMNs to meet future connectivity demands. This whitepaper highlights TEA Networks' dedication to advancing wireless networking technologies, offering a strategic framework for achieving scalable, efficient, and secure wireless infrastructures.
AUTHOR

Fatih KIR
Machine Learning Engineer
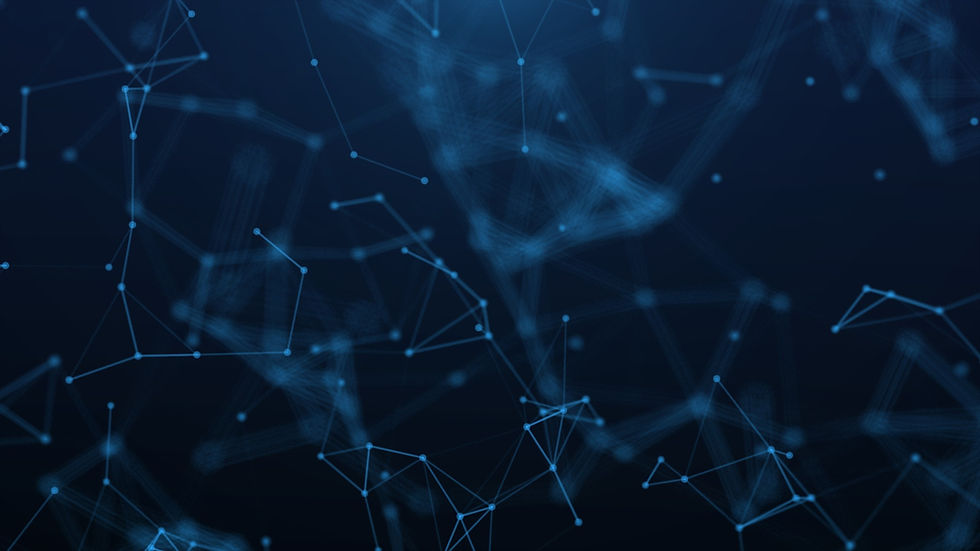
Introduction
In an era where connectivity is at the heart of technological advancements and business operations, wireless mesh networks (WMNs) emerge as a cornerstone for establishing robust, scalable, and efficient wireless infrastructures. These networks are pivotal in ensuring seamless communication, supporting a multitude of applications ranging from urban connectivity to emergency services. Despite their advantages, managing the complexities and dynamic nature of WMNs presents a myriad of challenges that can significantly affect their performance, security, and ability to scale.
The challenges inherent in wireless mesh networks are diverse and multifaceted. Interference, a prevalent issue, disrupts signal transmission, leading to degraded network performance and reliability. This is compounded by the need for optimal channel utilization, which is critical for maximizing bandwidth and ensuring smooth data transmission. Moreover, the detection of interference and the dynamic management of network channels require sophisticated tools and strategies to maintain network integrity.
This white paper introduces TEA Networks' innovative solution to these challenges: a channel utilization prediction framework designed to enhance the performance and reliability of wireless mesh networks. By leveraging advanced algorithms and comprehensive network analytics, our framework provides real-time insights into channel utilization, interference detection, and optimal network configuration. This proactive approach not only addresses the immediate challenges of network management but also sets the stage for scalable and efficient future network expansion.
Through this exploration, we aim to highlight the importance of sophisticated channel management and predictive analytics in overcoming the hurdles faced by wireless mesh networks. By doing so, TEA networks reaffirms its commitment to driving the evolution of wireless networking technologies, ensuring that organizations can leverage the full potential of their wireless infrastructure to support their operational needs and strategic goals.
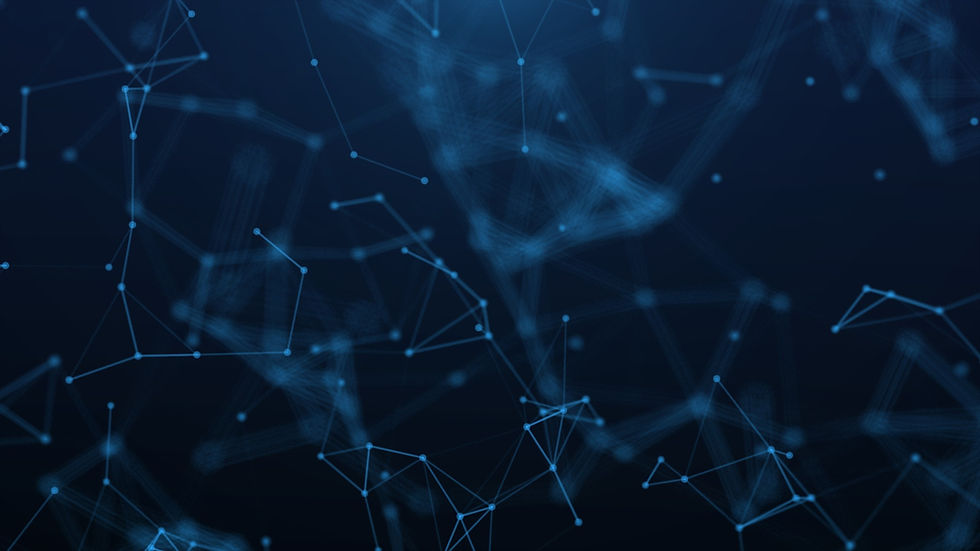
Feature Focus: Automated Channel Selection
Data Journey
The Automated Channel Selection feature in wireless mesh networks embarks on an intricate journey of data that begins with the diligent collection of interference metrics and the outcomes of continuous neighbor scans. This essential process is designed to optimize channel selection, ensuring the network's operational efficiency. At the core of this system is the network's controller, equipped with advanced monitoring software that plays a crucial role in the data collection phase.
From the onset, the controller is tasked with the continuous monitoring and recording of a wide array of data points. This includes interference patterns critical for channel selection, such as channel utilization and data retransmission rates. Such comprehensive monitoring is essential for understanding the network's dynamic environment and making informed decisions on channel allocation.
The transmission of this collected data to the cloud for analysis is facilitated through the use of the MQTT protocol, renowned for its efficiency in real-time data communication. Coupled with this is the employment of Protocol Buffers (protobuf) for data structuring. Protobuf plays a vital role in serializing the vast amounts of collected data into an optimized format. This combination not only ensures the integrity and confidentiality of the data but also addresses the complexity of managing a large volume of information.
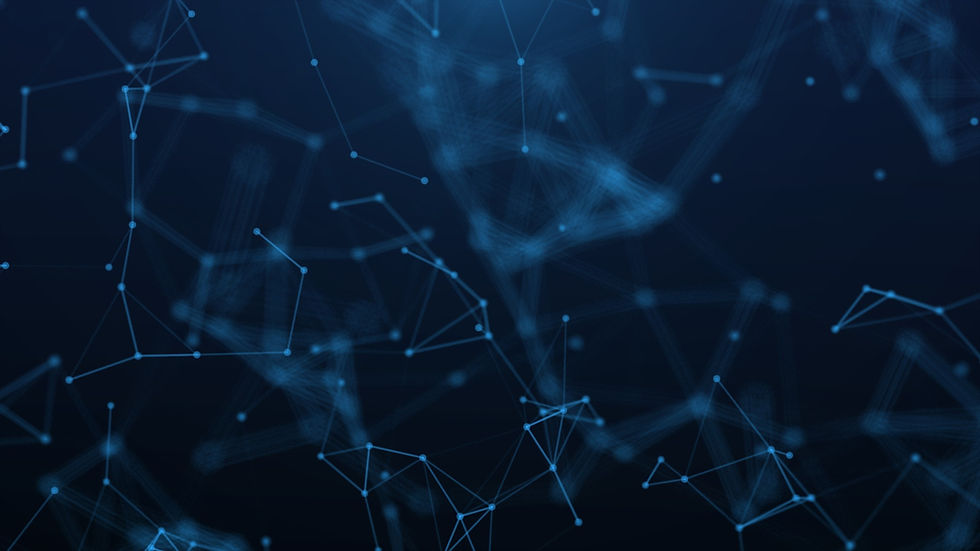
Design Approach
The design approach for the Automated Channel Selection feature is centered around enhancing network efficiency and scalability through intelligent, predictive analytics. This strategy involves a sophisticated process of analyzing interference data and leveraging advanced machine learning algorithms to forecast network conditions, particularly focusing on channel utilization metrics for the upcoming hour.
The foundation of this approach lies in the predictive capabilities of the system, which employs machine learning algorithms to anticipate potential interference scenarios before they adversely affect the network. To ensure rapid and accurate inferences for hundreds of thousands of households, Apache Spark's native implementations for machine learning algorithms are integrated. This choice leverages Apache Spark's powerful distributed computing capabilities, allowing for the processing of large datasets and the execution of complex algorithms at high speed. By analyzing historical data, the system can accurately predict high interference probabilities and assess the overall network health.
Upon identifying a high likelihood of interference, the system initiates a detailed evaluation of alternative channels. This evaluation is informed by comprehensive neighbor channel scan information, which provides insight into less congested channels that could serve as viable alternatives. The process is data-driven, ensuring that any channel switch is based on empirical evidence and aimed at optimizing network performance.
When the analysis concludes that a channel switch is necessary, the system generates and executes a channel selection action. This decision-making and execution process is designed to be seamless and minimally disruptive, ensuring that network performance is optimized without significant interruption to service.
The entire process, from data collection through to action execution, is supported by a robust data flow mechanism. Predictive analytics results and channel selection decisions are communicated through an integrated API, providing network administrators with access to crucial information. This transparency enables informed decision-making, allowing for swift adjustments to network configurations in response to predicted interference.
This design strategy, with its focus on predictive analytics, machine learning utilizing Apache Spark, and an adaptive framework, demonstrates a commitment to advancing the state of wireless mesh network management. By proactively addressing interference through automated channel selection, the feature ensures that the network remains resilient, efficient, and capable of meeting the demands of an ever-evolving digital landscape.

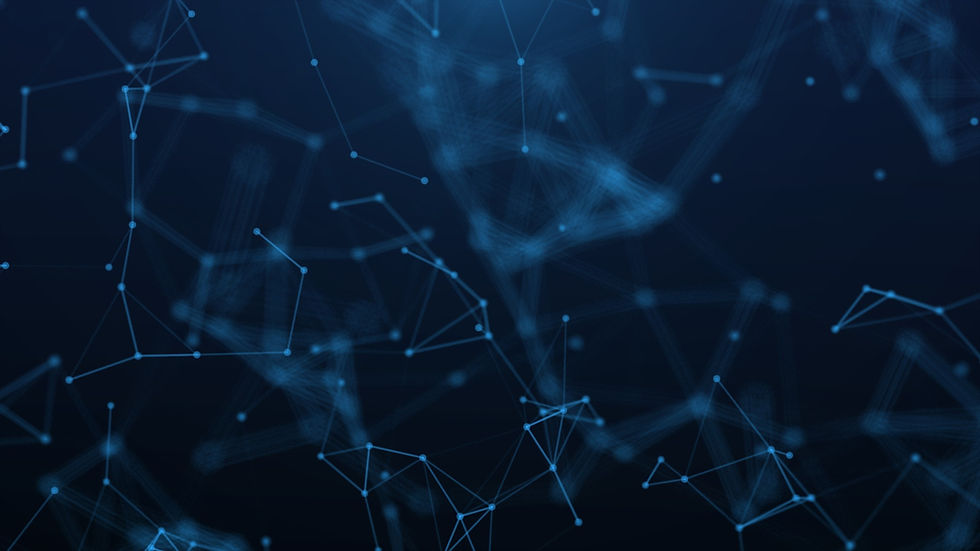
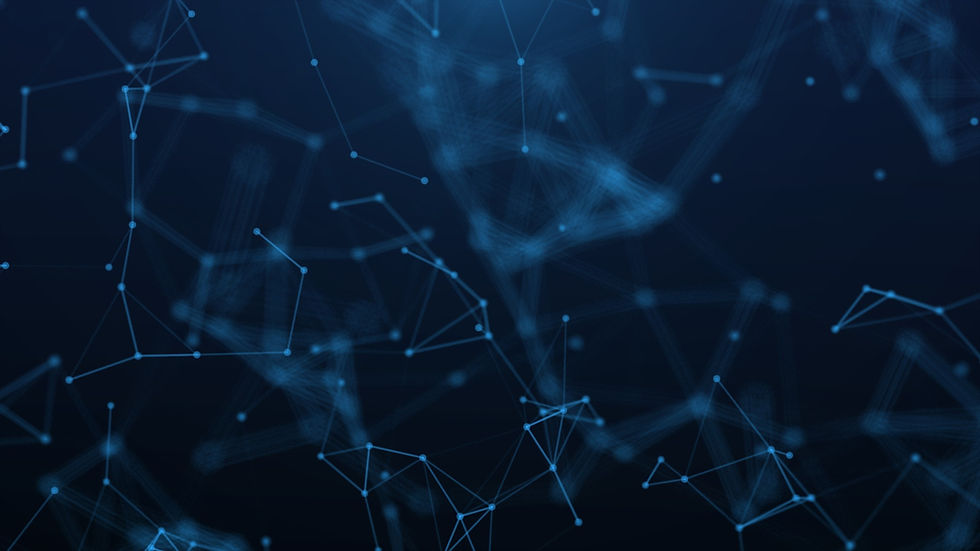
Data Pipeline Overview

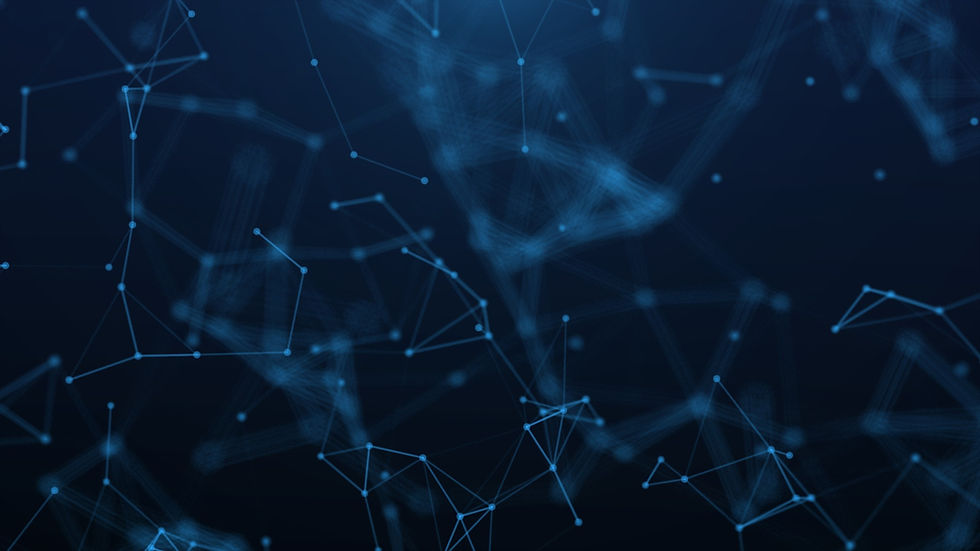
Conclusion
The implementation of the Automated Channel Selection feature represents a pivotal leap forward in the realm of wireless mesh network management. In an age where seamless digital connectivity forms the backbone of both business operations and everyday life, the imperative to sustain efficient, secure, and scalable networks has never been greater. Challenges such as interference and inadequate channel utilization stand as significant obstacles to achieving peak network performance.
This whitepaper has delineated TEA Networks' strategic response to these challenges, emphasizing a forward-thinking blend of predictive analytics and automated channel optimization. By employing state-of-the-art machine learning algorithms and capitalizing on Apache Spark's robust data processing capabilities, our framework not only predicts future interference scenarios but also dynamically adjusts channel settings to ensure optimal network function. This predictive approach, enriched with live network data, equips network administrators with the insights needed to make strategic decisions that boost network reliability and enhance end-user satisfaction.
The introduction of the Automated Channel Selection feature underscores TEA networks' dedication to pioneering advancements in network management technology. This tool not only addresses current interference issues but also scales to meet the future needs of evolving digital networks. Our methodology highlights the critical role that advanced network analytics and proactive management play in navigating the complexities of contemporary wireless mesh networks.
Looking forward, TEA networks is committed to pushing the boundaries of wireless networking technology further. Our ongoing investment in predictive analytics, machine learning, and design focused on user needs will continue to guide our innovation efforts. We are determined to develop solutions that not only resolve today's network challenges but also anticipate and adapt to tomorrow's demands.
In wrapping up, the Automated Channel Selection feature exemplifies our technological expertise and our vision for the next generation of wireless networking. It illustrates our resolve to tackle current challenges with future-ready solutions, setting new standards for connectivity, efficiency, and security in the digital age. With TEA networks, organizations can confidently navigate the complexities of digital connectivity, ensuring robust and seamless network performance for the future.
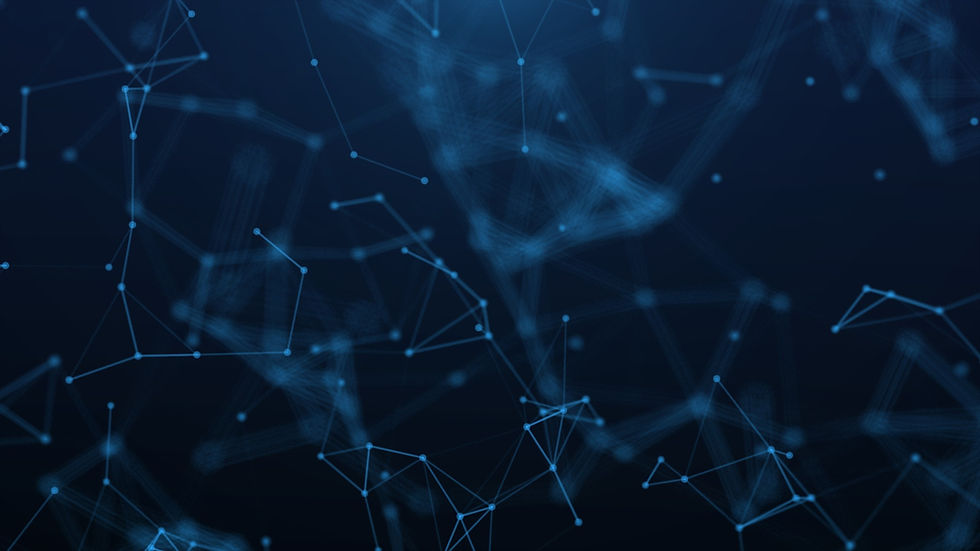
Integrating Automated Channel Selection
Integrating Automated Channel Selection into the network management system embodies a comprehensive strategy for optimizing wireless mesh network performance. This integration is crucial for maintaining network efficiency and stability by providing network administrators with actionable insights on an hourly basis.
At the heart of this integration is the display of predicted channel utilization data, which is meticulously analyzed and presented on the system's dashboard every hour. This predictive information offers a forward-looking view into the network's performance, enabling administrators to proactively manage network capacity and avoid potential bottlenecks. Similarly, results from continuous neighbor scans are presented alongside the utilization data, providing a detailed landscape of the network environment. This includes insights into the channels used by neighboring networks, which is vital for identifying less congested channels and potential interference sources.
When the system identifies a need for action—such as a channel switch to mitigate predicted high interference—this recommendation is also displayed prominently on the dashboard. Each suggested action is the result of a thorough analysis, including the evaluation of alternative channels based on neighbor scan data and the predicted network conditions. This level of detail and specificity in the recommendations allows network administrators to make informed decisions quickly, ensuring that the network can adapt to changing conditions with minimal disruption.
The user interface and reporting mechanisms of the Automated Channel Selection feature are designed for clarity and ease of use, ensuring that complex data and analytics are accessible and understandable. Network administrators are thus equipped with all the necessary information to grasp the current network state, the rationale behind each recommended action, and the potential impact of those actions on network performance. This integration not only simplifies the complex task of channel management but also empowers administrators with the tools to ensure an optimal user experience across the network.
This approach to integrating Automated Channel Selection represents an evolution in managing Wi-Fi networks, where predictive analytics and real-time data converge to create a dynamic, responsive network management environment. By leveraging detailed insights into channel utilization and neighbor activity, alongside clear, actionable recommendations, network administrators can maintain optimal network performance and resilience, even in the face of rapidly changing conditions. This proactive, data-driven strategy ensures that wireless mesh networks can continue to meet the demands of an increasingly connected and digital world.
