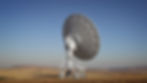
AnalyTEAcs'
Daily Anomaly Detection
Daily Anomaly Detection
ABSTRACT
In an era where Wi-Fi networks are vital to business operations, managing their complexity and vulnerability to threats is paramount. This white paper presents an innovative solution—TEA Networks' AnalyTEAcs, which employs a Daily Anomaly Detection feature to identify and respond to network irregularities. By harnessing data from various sources, the tool utilizes advanced algorithms to detect anomalies, improving network integrity and operational efficiency. This study outlines the challenges of Wi-Fi management, the journey and treatment of data through TEA's tool, and the resulting benefits. The daily monitoring provides a robust defense, enhancing both security and performance. We conclude with the future trajectory of AnalyTEAcs, emphasizing continuous improvement and the integration of sophisticated analytics for proactive network management.
AUTHORS
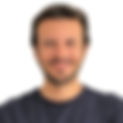
Kerim Aytek Tok
Co-Founder, CEO

Kayra Bilgin
Data Scientist
Table of Contents
4 Introduction
5 Feature Focus: Daily Anomaly Detection
5 Journey of Data
5 Design Approach
6 Integrating Daily Monitoring
7 Data Pipeline Overview
8 Conclusion

Introduction
In the era of digital transformation, Wi-Fi networks serve as the critical infrastructure underpinning a wide array of business operations, communications, and essential services. Getting these networks to work smoothly is essential for any organization's success and its overall efficiency. However, the management of these complex and expansive networks is full of challenges that can significantly impact performance, security, and scalability of the network.
These challenges are multifaceted and dynamic in nature. Modern Wi-Fi networks are confronted with a spectrum of issues that test their robustness and adaptability. Security threats, such as cyber attacks and vulnerabilities in Wi-Fi protocols, pose constant risks, threatening the integrity and confidentiality of network data. Network performance is also a critical area, with issues like bandwidth management and interference directly impacting the reliability and consistency of services. As organizations grow, their networks must scale correspondingly, a task complicated by the burgeoning demand for connectivity and the rapid evolution of technology. Additionally, ensuring a seamless user experience necessitates efficient access control and uninterrupted connectivity.
This white paper delves into how AnalyTEAcs, Wi-Fi Management Tool of TEA networks, does daily anomaly detection, which is just one of its innovative solutions to these multifaceted challenges. The ability to detect and respond to network anomalies is an important factor in maintaining the integrity and efficiency of these networks.
4
Feature Focus: Daily Anomaly Detection
Data Journey
The basis of Daily Anomaly Detection within Wi-Fi networks lies in the extraction and analysis of event messages generated from Wi-Fi devices. This data is crucial in identifying and troubleshooting various network anomalies and ensuring robust and secure Wi-Fi management.
Each Wi-Fi device, managed by the TEApot controller, is embedded with sophisticated software designed to continuously monitor and record a variety of critical events. These events range from system-level occurrences like controller restarts and memory shortages to network-specific incidents such as node disconnections or internet access issues.
The process of transmitting event data from the Wi-Fi devices to the cloud is streamlined, utilizing the MQTT protocol. The data is structured using protobuf (Protocol Buffers), which provides an efficient format for serializing structured data. This combination of MQTT for transmission and protobuf for data structuring provides that the data's integrity and confidentiality are maintained, while also simplifying the complexity of the data transmission process. The TEApot controller, serving as the central hub in this architecture, efficiently manages this data flow.
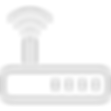
TEA device
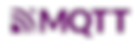
machine to machine network protocol for message queue
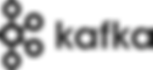
distributed data store and stream-processing platform
Design Approach
The design approach in the development of Daily Anomaly Detection feature has been prepared to enable efficiency and scalability. The basis of our design approach is comprehensive analysis and integration of event data from a wide range of sources in Wi-Fi networks. This integration is crucial to capture a complete picture of network behavior and enables the detection system to identify anomalies.
Advanced machine learning algorithms and analytics are integrated into an adaptive architectural framework in the design of Daily Anomaly Detection feature. These selected and fine-tuned algorithms can uncover patterns and anomalies in complex network environments that might be missed by conventional methods. Complementing this is the system's adaptable architecture designed to manage the evolving demands and increasing complexity of modern Wi-Fi networks. This scalability ensures consistent effectiveness in anomaly detection because models can perform better with larger data.
Anomalies detected from the data analytics process are written to Kafka and transferred to the database from there. These data are displayed in their appropriate places on TEA dashboard via API.

data analytics
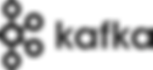
distributed data store and stream-processing platform

PostgreSQL database

API

TEA dashboard
5
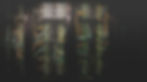
Integrating Daily Monitoring
The integration of Daily Anomaly Detection in AnalyTEAcs epitomizes a holistic approach to network monitoring. Daily Anomaly Detection feature provides a broad, aggregated analysis of network activities over a 24-hour period, identifying trends and evolving patterns.
AnalyTEAcs' user interface and reporting mechanisms are designed to not only be user-friendly but also highly detailed in presenting data insights. When an anomaly is detected, the system specifically identifies which elements of the network are affected and supports these findings with relevant data analysis. This level of detail allows network administrators to understand precisely why an event is classified as an anomaly, offering a clear basis for response actions. This synthesis of daily monitoring represents an advanced, adaptive approach to managing Wi-Fi networks.


6
Data Pipeline Overview




7
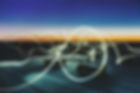
Conclusion
The development and implementation of Daily Anomaly Detection feature in AnalyTEAcs mark a significant advancement in the realm of Wi-Fi network management. Daily Anomaly Detection offers a comprehensive, aggregated view of network health over extended periods, allowing for the identification of long-term trends and patterns. This feature forms a robust framework that enhances network security, optimizes performance, and ensures a proactive response to potential risks. It embodies the perfect blend of strategic foresight, crucial in today's fast-paced and ever-evolving digital landscape.
Looking ahead, TEA networks is committed to continuous innovation and improvement in its AnalyTEAcs. While the project's design is elementary, it is planned to optimize resource usage and is scalable for future enhancements. These improvements could involve deeper time-series analysis, refining the existing models through more trials and parameter fine-tuning, and implementing supervised learning algorithms once the system accumulates sufficient data for self-learning. The ultimate goal is to develop a model that self-adjusts daily, delivering accurate and detailed insights. This vision for the future encompasses the integration of more advanced analytics, the exploration of predictive modeling, and the potential adoption of AI-driven approaches to anticipate and adapt to the evolving needs of Wi-Fi networks.